Microscopy: Deep learning improves microscopy images—without system adjustments
Is it possible to significantly boost an optical microscope’s performance without making changes to its hardware or design, or even requiring user-specified postprocessing?
Yes, say researchers at the University of California, Los Angeles (UCLA). Aydogan Ozcan, associate director of the UCLA California NanoSystems Institute and the Chancellor’s Professor of Electrical and Computer Engineering led the team, including postdoctoral scholar Yair Rivenson and graduate student Yibo Zhang, to demonstrate that a deep neural network can generate an improved version of a standard microscope image in <1 s using only a laptop computer. Remarkably, the network can match the capabilities of a high-numerical-aperture (NA) microscope objective in terms of resolution, and surpass it in terms of field of view (FOV) and depth of field (DOF).
Deep learning microscopy
A deep neural network represents a structured approach to deep learning—a machine-learning method that automatically analyzes signals or data using stacked layers of artificial neural networks. One such network type, the deep convolutional neural network (CNN), represents a fast-growing field of research with numerous applications. Each layer within a CNN includes a nonlinear operator and a convolutional layer with kernels (filters) that can learn to perform tasks using supervised or unsupervised machine-learning approaches.
Deep-learning-based microscopy begins with the statistical relationship between low- and high-resolution imagery that is necessary for transforming images and for training a CNN (see figure). Traditional image processing normally uses spatial convolution operation followed by an under-sampling step for this purpose, which transforms a high-resolution, high-magnification image to a low-resolution, low-magnification depiction. Instead, though, the researchers have proposed a CNN framework that trains multiple layers of artificial neural networks to statistically relate low- and high- resolution images for input and output, respectively.
To train and test the framework, the UCLA team used a bright-field microscope with spatially and temporally incoherent broadband illumination, a setup that makes it relatively difficult not only to produce an exact analytical or numerical model of light-tissue interaction, but also to form the corresponding physical image. This makes the relationship between high- and low-resolution images more difficult to model or predict.
The researchers trained the CNN with a lung tissue model, and blindly tested it on various tissue samples (kidney and breast) as well as a resolution test target. They used a oil-immersed 40x 0.95 NA objective lens with a 0.55 NA condenser. Subsequently, they applied the CNN to quantify the deep neural network’s effect on the spatial frequencies of the output imagery.
Evaluation and application
To evaluate the modulation transfer function (MTF), the researchers calculated contrast among the resolution test target’s various elements. This experimental analysis became the basis for comparing MTFs for input and output images of the deep neural network trained on lung tissue. Despite being trained on tissue samples imaged with a 40x 0.95 NA objective lens, increased modulation contrast is evident in a large portion of the output image’s spatial frequency spectrum (especially at the high end), while also resolving a period of 0.345 μm.
The researchers report that their deep learning approach takes, on average, approximately 0.69 s/image with a FOV of about 379 × 379 μm using just a laptop computer. It works with a single image captured using a standard optical microscope—nothing extra is necessary. They report that with appropriate training, the entire framework (and derivatives thereof) might be effectively applied other optical microscopy and imaging modalities (for example, fluorescence, multiphoton, and optical coherence tomography) and even assist in designing computational imagers with improved resolution, FOV, and DOF.
REFERENCE
1. Y. Rivenson et al., Optica, 4, 11, 1437-1443 (2017).
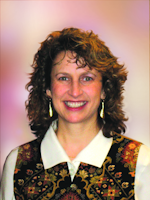
Barbara Gefvert | Editor-in-Chief, BioOptics World (2008-2020)
Barbara G. Gefvert has been a science and technology editor and writer since 1987, and served as editor in chief on multiple publications, including Sensors magazine for nearly a decade.