AI and microscopy may revolutionize study of cells, molecule behavior
Artificial intelligence (AI) can identify patterns and relationships in data that may be difficult for humans to discern, so University of Gothenburg researchers are combining microscopy with AI to help spot subtle changes in biological motion (see video).
“AI has become increasingly used to analyze images obtained from digital microscopy, following the deep-learning revolution,” says Jesús Pineda, a doctoral student at Gothenburg and first author of research published in Nature Machine Intelligence. “This has marked a shift from conventional microscopy analysis methods that rely on human interpretation and manual processing.”
It can be a reliable method for characterizing motion patterns, as well, Pineda says.
AI’s ability to process large amounts of data quickly and accurately makes it a valuable tool for researchers who need to analyze extensive datasets or for practitioners who require real-time decisions based on complex data.
The team’s new AI/deep learning method combines graph theory and neural networks to extract dynamic information reliably and accurately from microscope videos. The technique uses a particle graph—a mathematical structure that represents relationships between elements—to model the movement and interactions of particles in a video.
“Graphs can be readily understood by portraying them as a social network where users—the elements in the graph—interact and influence each other’s behavior directly or indirectly,” says Pineda. “In a similar manner, our AI creates a social network of particles that captures complex interplay and relationships.”
Living cells move constantly, at different speeds and in every conceivable direction, which makes it difficult to precisely and clearly film and document their biological processes under a microscope. The team’s method provides researchers with a closer look at these processes and the complex relationships of particles and elements as they interact and influence each other’s behavior (see Fig. 1).
Significant developments in optical microscopy during the past two decades allow researchers to study living systems with unprecedented detail at various scales by collecting enormous amounts of data from microscope videos, Pineda says, but developing algorithms to quickly analyze it remains a challenge.
“The diversity in biological motion is a significant contributor to the intricacy of the analysis,” he says. “The movement of cells, for example, exhibits a high degree of variability depending on factors such as cell type and environmental cues, suggesting that a universal biological motion analysis method may be unattainable. That is, until now.”
Their AI technique uses the information in a particle graph to adapt to different dynamics. It can solve multiple tasks presented in various “challenging experimental scenarios,” Pineda says (see Fig. 2). “For example, our AI allows scientists to reconstruct the path that individual cells or molecules follow as they move to accomplish biological function.”Such information is crucial in applications such as cancer research and drug development, he says. By following the movement and behavior of cells, the effectiveness of different drugs can be tested so “we can see how well they work as potential treatments for cancer,” he says.
“This approach offers new perspectives on drug development by providing researchers with a more efficient and accurate method to analyze the motion of molecules and cells,” Pineda says, noting that it could lead to the development of more effective treatments for not only cancer, but a wide range of other diseases.
The team’s technique has also become a key enabling technology to help researchers estimate any dynamic aspect of particles in situations where other methods would not be effective—such as a consequence of high object density or nonoptimal data acquisition. It could potentially revolutionize the biotech industry as well as biological motion analysis by enabling high-precision and accurate examination of spatiotemporal features.
“With its ability to learn from large amounts of data and make accurate predictions based on past examples, our AI offers several advantages over traditional methods,” Pineda says. “And by enabling more efficient analyses and ground-breaking discoveries, our new method is set to play a critical role in shaping the future of biotech research and development.”
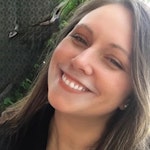
Justine Murphy | Multimedia Director, Digital Infrastructure
Justine Murphy is the multimedia director for Endeavor Business Media's Digital Infrastructure Group. She is a multiple award-winning writer and editor with more 20 years of experience in newspaper publishing as well as public relations, marketing, and communications. For nearly 10 years, she has covered all facets of the optics and photonics industry as an editor, writer, web news anchor, and podcast host for an internationally reaching magazine publishing company. Her work has earned accolades from the New England Press Association as well as the SIIA/Jesse H. Neal Awards. She received a B.A. from the Massachusetts College of Liberal Arts.