Developed by researchers led by the Broadband Infrared Diagnostics team at Ludwig-Maximilian University München and the Max Planck Institute of Quantum Optics, both in Germany, a new health screening system uses infrared spectroscopy—a common technique in medical diagnostics—integrated with machine learning to reveal information about the molecular profiles of multiple complex biofluids and ultimately health conditions via a single measurement from just one drop of blood (see video).
“While the concept of analyzing molecules of human blood for clinical diagnostics is well established, our method leverages infrared molecular spectroscopy for robust and reproducible human blood plasma fingerprinting,” says Dr. Mihaela Žigman, research group leader of the Broadband Infrared Diagnostics team.
Looking back
The new approach stems from previous work the team began that involves Fourier transform infrared (FTIR) spectroscopy, a method that has long been used in experimental studies for disease detection. In their initial study, the team discovered that infrared molecular fingerprints are not only unique to each individual, but also remain consistent over time.
“This finding suggested that our approach held promise for clinical diagnostics in monitoring human health,” Žigman says.
They first applied their FTIR spectroscopy method to cancer diagnostics, comparing individuals with one of four common cancers with a control group that exhibited similar characteristics but without malignancy. The researchers demonstrated that their approach involving blood serum or plasma could detect severe abnormalities, including cancers.
Moving forward
In their more recent work—detailed in Cell Reports Medicine—the team expanded their focus to explore whether the same spectroscopic approach could be used to identify those with various common conditions within a naturally heterogeneous population.
Collaborating with researchers at Helmholtz Center Munich (Germany), who had previously conducted a population study involving random (non-matched) adults who participated in medical evaluations including blood analysis, provided the team a unique opportunity to test whether IR fingerprinting could detect not just cancer but also a range of common medical conditions.
“A key aspect of our approach was the integration of machine learning,” Žigman says. “We combined the medical data from the studied individuals with their newly measured spectroscopic IR fingerprints of blood plasma and used this combined dataset to train algorithms capable of identifying both single and combined conditions.”
With thousands of data points available, the team trained the algorithms effectively and could validate their accuracy on unseen datasets.
The primary advantage of the new approach lies in its ability to characterize an individual's health through a single, noninvasive, cost-efficient measurement, Žigman explains. Conventional blood-based diagnostic tests often require multiple rounds to arrive at a diagnosis, each targeting specific conditions or markers.
“Our method enables the simultaneous detection of multiple molecular contributions and conditions in a molecule-agnostic and condition-agnostic manner, making it both efficient and comprehensive,” Žigman says.
Goals
The team aims to ultimately improve the characterization of human health using this simple blood-based spectroscopic measurement.
“We want to detect diseases early, giving us the opportunity to manage or even prevent their development,” Žigman says. “I wish to make a significant impact on clinical diagnostics. This includes not only enhancing the early detection of diseases, but also gaining a deeper understanding of what it means to be healthy.”
Beyond detecting the presence of health conditions, this approach could also potentially be used to test for the absence of a disease, Žigman says, “offering a novel way to monitor overall health that could change preventative healthcare.”
By efficiently characterizing and understanding molecular profiles, the team may be able to develop algorithms to identify specific health and disease phenotypes.
FURTHER READING
T. Eissa et al., Cell Rep. Med., 5, 7, 101625 (2024); https://doi.org/10.1016/j.xcrm.2024.101625.
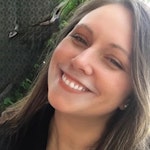
Justine Murphy | Multimedia Director, Digital Infrastructure
Justine Murphy is the multimedia director for Endeavor Business Media's Digital Infrastructure Group. She is a multiple award-winning writer and editor with more 20 years of experience in newspaper publishing as well as public relations, marketing, and communications. For nearly 10 years, she has covered all facets of the optics and photonics industry as an editor, writer, web news anchor, and podcast host for an internationally reaching magazine publishing company. Her work has earned accolades from the New England Press Association as well as the SIIA/Jesse H. Neal Awards. She received a B.A. from the Massachusetts College of Liberal Arts.