Millions of optical coherence tomography (OCT) images are acquired each year, providing anatomical insights to diagnose numerous medical conditions, including diabetic macular edema (DME). Deep learning is a form of artificial intelligence (AI) in which large amounts of data are processed to combine key factors and predict an output. Deep learning needs an ample database to reach conclusions. OCT generates abundant data, but needs more-efficient data analysis. It’s a natural fit, and an area of burgeoning activity (see figure).
Applying AI to the eye
Convolutional neural networks (CNNs) are a class of AI that enables deep learning. CNNs perform two separate, but related, tasks in image processing: segmentation and classification. Segmentation is the identification of related pixels in an image to define a region or regions—for example, identifying where the optic nerve head appears in an image of the retina. Classification is the identification of an object or objects belonging to a predefined group, such as labeling the optic nerve head as normal or abnormal.
René Werkmeister is a researcher at the Medical University of Vienna (Austria). His team used deep learning to segment ophthalmic OCT images in order to recognize the tear meniscus, which is the layer of liquid present as the eyelid traverses the surface of the eye. Patients suffering from dry-eye disease have abnormal meniscus geometries, quantifiable with measures such as tear meniscus volume, height, or radius of curvature. These measurements need the tear meniscus to be separated from other regions in an image. This is time-consuming and subject to operator error, so Werkmeister’s team applied CNNs to the problem.
They compared three approaches: Their previously developed threshold identification routine based on standard image processing tools; a one-phase neural network that segments the tear meniscus from a large OCT image; and a two-phase neural network that first selects a region of interest and then segments the tear meniscus from that smaller region. Both of the CNN approaches were as accurate as the standard image processing, and significantly faster. Hannes Stegmann, a member of Werkmeister’s team, says the speed improvement is important “primarily for the analysis of clinical trials, where large volumes of data are acquired, requiring long processing times when analyzed by conventional algorithms.”
Deep learning, deeper in the eye
Sina Farsiu, director of the Vision and Image Processing Laboratory at Duke University (Durham, NC), leads a team looking to optimize treatment of diabetic retinopathy (DR). Advanced DR blocks existing retinal blood vessels and triggers formation of new ones. The current standard of care is to treat every patient with antivascular endothelial growth factor (anti-VEGF) drugs, and then see if the patient responds. Many DR patients will not, and that, the team notes, is “costly and burdensome for both patients and physicians.” Farsiu’s goal was to classify responsive and unresponsive patients by analyzing a single pre-treatment OCT image set.
They collected a database of images from 127 patients, all with diabetic macular edema, which is fluid leakage that thickens the retina and degrades vision. All patients were treated with one of the standard anti-VEGF drugs at four- to six-week intervals. After three treatments, OCT images were again acquired, reflecting the clinical practice of evaluating patients’ response at that time. Members of the study group were classified as “responsive” if the retinal thickness decreased by at least 10%, and “nonresponsive” otherwise. Eighty of the patients were responsive and 47 nonresponsive, consistent with percentages in the general population.
Farsiu’s team created a CNN architecture with six “attention blocks,” which are essentially elements that allow the network to weight “interesting” image areas more heavily. They compared the CNN performance against that of several popular learning architectures. Their architecture significantly outperformed the alternatives, with 87% precision and 85% specificity. Performance for very responsive and very unresponsive patients was even better. The team concluded that “the ability to accurately select highly responsive and very poorly responsive patients prior to treatment would be beneficial for practicing physicians and potentially for subject selection in clinical trials of novel therapies for DME.”
Challenges remaining
Although deep learning can optimize clinical efficiency, challenges remain. Recent review papers highlight the need for larger and more diverse datasets, along with additional routines to make CNNs more robust to machine- or population-specific artifacts. Daniel Shu Wei Ting, a professor in ophthalmology at the Duke University/National University of Singapore, and his collaborators are troubled by the lack of transparency in deep learning. CNNs operate on highly abstracted levels of data, making decision-making opaque. They note that “clinicians and patients are still concerned about AI and deep learning being ‘black-boxes’…[and understanding] the underlying features through which the algorithm classifies disease…is important to improve physician acceptance.”
In a separate review, Ting and other colleagues repeat those concerns, and summarize the current state: “Although deep learning and OCT have individually revolutionized ophthalmology, optimizing the combined technologies will be integral to accelerating progress in the field.”
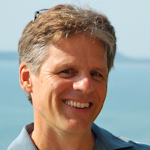
Richard Gaughan | Contributing Writer, BioOptics World
Richard Gaughan is the Owner of Mountain Optical Systems and a contributing writer for BioOptics World.