Thermal Imaging: Software algorithms and thermal imaging combine to recognize faces in the dark
Facial recognition continues to be challenging, but is advancing in large part because of specialized software algorithms that take clues from the surrounding environment for face detection and then analyze and classify facial features that aid in the identification process against a known database of visible-light images. Researchers at the U.S. Army Research Laboratory (ARL; Adelphi, MD), however, are taking facial recognition a step further by using thermal imaging and artificial intelligence to also perform facial recognition in low-light conditions or at night.1
Motivated by nighttime surveillance and other combat operations, the ARL technique takes conventional thermal or polarimetric thermal images of a person’s face and seeks to synthesize a visible-like image that in turn can be matched to generic visible-light images stored in biometric face databases or watch lists using standard commercial-off-the-shelf (COTS) or open-source facial recognition software.
Global and local features
The ARL synthesis approach uses domain adaptation techniques based on deep neural networks. Synthesis begins with a nonlinear regression model that maps features extracted from a thermal face image into the corresponding visible domain feature representation, and then reconstructs a face in visible image space using convolutional neural networks (CNNs) through a multi-region optimization procedure.
This optimization procedure attempts to preserve the global shape of the entire face as well as the local details around fiducial regions such as the eyes, nose, and mouth. While this technique can be applied effectively to conventional intensity-only thermal imagery, the ARL team found that incorporating polarization-state information further improves the fidelity of reconstructed visible face images.
In an experiment on a database containing both visible and thermal images from 60 unique individuals, it was found that the ARL algorithm improved facial recognition performance by about 3% for conventional thermal images and about 5% when polarimetric thermal images were used as the input for synthesis, compared to another state-of-the-art deep-neural-network-based approach. Apparently, the ARL method is able to capture more of the structural information of the face, which is critical to a robust facial recognition process.
REFERENCE
1. B. S. Riggan, N. J. Short, and S. Hu, arXiv:1803.07599v1 [cs.CV] (Mar. 20, 2018); https://arxiv.org/pdf/1803.07599.pdf.
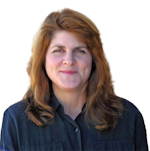
Gail Overton | Senior Editor (2004-2020)
Gail has more than 30 years of engineering, marketing, product management, and editorial experience in the photonics and optical communications industry. Before joining the staff at Laser Focus World in 2004, she held many product management and product marketing roles in the fiber-optics industry, most notably at Hughes (El Segundo, CA), GTE Labs (Waltham, MA), Corning (Corning, NY), Photon Kinetics (Beaverton, OR), and Newport Corporation (Irvine, CA). During her marketing career, Gail published articles in WDM Solutions and Sensors magazine and traveled internationally to conduct product and sales training. Gail received her BS degree in physics, with an emphasis in optics, from San Diego State University in San Diego, CA in May 1986.