With new differential detection scheme, all-optical diffractive neural networks get performance boost
A new paper in Advanced Photonics, an open-access journal co-published by SPIE and the Chinese Laser Press, demonstrates distinct improvements to the inference and generalization performance of diffractive optical neural networks.1 Such networks are potentially valuable for efficient machine learning, opening the door to lower-cost versions of the many artificial-intelligence (AI) and machine- and deep-learning applications that are springing up across the digital world.
One of the key improvements discussed in the paper, titled "Class-specific differential detection in diffractive optical neural networks improves inference accuracy," incorporates a differential-detection scheme combined with a set of parallel-operating diffractive optical networks in which each individual network in the set is specialized to specifically recognize a sub-group of object classes. The fact that light intensity can't dip below zero has restricted some operations in the past, but the differential measurement technique gets around this hindrance to broaden the capabilities of the diffractive optical neural network.
According to SPIE Fellow Aydogan Ozcan of the University of California, Los Angeles (UCLA), and one of the paper's authors, these results "provide a major advancement to bring optical neural network-based low-power and low-latency solutions for various machine-learning applications."
This latest research is a significant advance to Ozcan's optical machine-learning framework: the finessing of this technology is especially significant for recognizing target objects more quickly and with significantly less power than standard computer-based machine learning systems. Ultimately, it may provide major advantages for autonomous vehicles, robotics, and various defense-related applications, among others.
These latest systematic advances, in diffractive optical network designs in particular, have the potential to advance the development of next-generation, task-specific, and intelligent computational camera systems.
Source: https://www.eurekalert.org/pub_releases/2019-08/ssfo-adn081219.php
REFERENCE:
1. Jingxi Li et al., Advanced Photonics, 1(4), 046001 (2019); https://doi.org/10.1117/1.AP.1.4.046001.
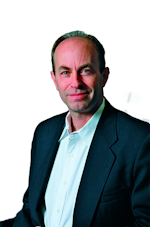
John Wallace | Senior Technical Editor (1998-2022)
John Wallace was with Laser Focus World for nearly 25 years, retiring in late June 2022. He obtained a bachelor's degree in mechanical engineering and physics at Rutgers University and a master's in optical engineering at the University of Rochester. Before becoming an editor, John worked as an engineer at RCA, Exxon, Eastman Kodak, and GCA Corporation.