A good race is exhilarating, especially when competition is fierce and the lead changes regularly. As spectators it’s easy to get caught up in the excitement, watching as the competition pivots, making strategic moves to build an advantage or take the lead. Of course, when rooting for one participant’s success, it’s easy to focus on the actual event without considering everything happening in the background. And race day is little more than the culmination of tireless hours of work.
Motorsports are prime examples of an environment where spectators typically have a cursory idea at best of what happens behind the scenes. There is a team behind each driver, and not just the pit crew. Engineers are often located trackside, tasked with making split-second decisions—decisions with real consequences. Beyond experience, making those decisions requires insight into what is happening on the track such as course-related difficulties, vehicle issues, as well as how driver decisions are impacting performance. It’s a complex equation.
With hopes of gaining an edge on the competition, Andretti Autosports (Indianapolis, IN) entered a multi-year, multi-million-dollar partnership with Zapata Computing (Cambridge, MA) to build cutting-edge data science and machine-learning solutions (see figure).
“We were hired to do a specific job, which is to help them win races,” says Zapata CTO and co-founder Yudong Cao. “We need to get them the necessary data, whenever and however they need it. We need to be able to provide them with things they can’t figure out in real-time given the pace of the race, or information they don’t necessarily find intuitive, yet can be tremendously helpful if we can uncover it from data.”
There are also different timescales in which Andretti would like to carry out its analytics, each with different learning opportunities. “Opportunities exist within each of the many dimensions—the tech stack (including heterogenous, hybrid multi-cloud environments, on-prem and edge computing) is a dimension, the timescale is a dimension, and algorithm solutions are another dimension,” Cao says. “This is true when they’re racing, when they’re offline from the racing weekends, and when they’re analyzing their season.”
Success requires very tight conversations with Andretti, so Zapata understands the whole racing activity. “This is the biggest challenge we’re looking to overcome. It’s the kind of challenge facing most AI/ML solutions,” Cao says. “It’s one thing to offer a cool algorithm to solve a math puzzle where you drop data into a container and the rest is automated. But to really build something useful requires looking at how they’re thinking about it themselves and how their intuitions are formed based on past experiences.”
Yet, Cao is a firm believer that before there’s machine learning, there needs to be human learning. This human learning has come from sitting down with Andretti engineers in the race command center, in the pits, at their Indianapolis headquarters, in the machine shops—the list goes on and on. “Without becoming part of their team and rolling with them at every race, we can’t understand their world,” he says. “We can build all the software in the world, but at the end of the day, they’re probably going to use a negligible fraction of it. And that’s not the goal we have here. The goal is that everything we build must fit their needs like a glove.”
Zapata has already identified key focus areas. “While we have racing enthusiasts, from the perspective of a sports fan, we started from zero understanding about the inner workings of motor racing. Today, we have specific use cases along the line of machine learning and data engineering—opportunities mutually agreed on to be of high impact immediately,” he says.
The quantum race
Of course, not all races move as fast as an Indy car. Some, like the race to build quantum computers ready for commercialization, better resemble an ultramarathon run by sloths. After all, quantum is the technology that’s been “five years away” for decades now. Hopefully the finish line is on the horizon.
While numerous approaches exist, photonics-based technologies are in a good position to win. With classic photonics, using wave mechanics with a photon carrying out arithmetic operations, companies like Ayar Labs building photonic interconnects and Intel building the photonic integrated circuits are ideal for addressing what Cao calls fascinating algebra calculations. “These are worth looking into from a standpoint of improving some of the machine-learning applications because it involves a lot of these fundamental linear algebra operations,” he says.
There’s also a process known as quantum photonics, which takes advantage of the properties of the photon as a quantum-particle-enabling behavior manipulation to process information. “While different from the mainstream quantum computing, it is a promising direction in terms of demonstrating postclassical behavior,” Cao says. There are two instances in the world of “quantum supremacy,” one demonstrated by the Chinese Academy of Science in 2020. And one demonstrated this year by Xanadu, where it’s possible to build quantum photonic devices to produce postclassical sampling ability and address applications such as generative modeling.
There are also efforts to use quantum photonics for mainstream gate model quantum computing. “It’s alongside superconducting qubits and trapped ions, it’s another modality and we don’t know whether it is going to be a winner in that race. Only time will tell,” Cao says.
Indy application
For Andretti, quantum is part of the portfolio of next-generation computing resources alongside GPU, FPGA, and other ASICs. Quantum provides extra enhancements for machine-learning solutions, and there are several stages between where Zapata is now and when it will be ready to deploy quantum resources.
Zapata is initially using a quantum-inspired data structure built to run on a quantum device. “On the engineering side, we need to set up infrastructure to make sure that we cannot only integrate quantum resources, but heterogeneous computing resources whether it’s a specialized chip for training AI models, or other kinds of dedicated ASICs,” he says. “Quantum is a special kind of computing resource, and we’re setting the foundation for it to boost the machine learning model we’re building.”
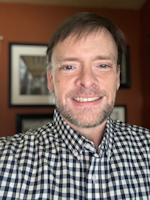
Peter Fretty | Market Leader, Digital Infrastructure
Peter Fretty began his role as the Market Leader, Digital Infrastructure in September 2024. He also serves as Group Editorial Director for Laser Focus World and Vision Systems Design, and previously served as Editor in Chief of Laser Focus World from October 2021 to June 2023. Prior to that, he was Technology Editor for IndustryWeek for two years.
As a highly experienced journalist, he has regularly covered advances in manufacturing, information technology, and software. He has written thousands of feature articles, cover stories, and white papers for an assortment of trade journals, business publications, and consumer magazines.