Can sound waves be used to create and mediate optical neural networks? Neural networks are inspired by the brain and use synapses and electrical or chemical signals between them. Within optical neural networks, signals are carried via light.
Attempts to create optical neural networks aren’t new—think structures on photonic chips or diffractive neural networks made of phase plates. But Birgit Stiller, head of the Quantum Optoacoustics research group at MPL and a full professor at Leibniz University Hannover in Germany, is trying to pair acoustic waves with light to create the building blocks for an optical neural network that enables a new type of computing architecture (see video).
“Our dream is to build a complete neural network operating within the photonic domain, managed by sound waves,” Stiller says. “This type of network can work inside a standard optical fiber commonly used for telecommunications.”
Researchers are often “surprised by the amount of information and the properties of light that can be captured within an acoustic wave,” Stiller says. “Acoustic waves are macroscopic, slow, dissipative objects with a ‘low’ frequency (compared to light)—photonics people tend to view them as banal and bulky objects.”
But a pure light source can be coupled with such a sound wave to transfer information back and forth. Sound waves carry the optical phase, its orbital angular momentum, information about its optical wavelength, and even its polarization in particular circumstances.
Stiller’s team recently created an optoacoustic recurrent operator (OREO)1 as the basic building block for a recurrent neural network (commonly used for sequential data processing). It’s built inside optical fiber, so it doesn’t require any extra fabricated structures and can be controlled by external light pulses.
Building blocks
To date, Stiller’s group has created three initial building blocks for optoacoustic neural networks.
How does the first one, OREO, work? Acoustic waves enable one computation step to connect to subsequent ones. “This means one computational step can suddenly also process the context of the data—not only one data point,” Stiller says. “If you have the same words within a sentence, but switch the order, you need contextual information to capture its meaning. ‘The elephant carries the bird’ is quite different than ‘the bird carries the elephant.’”
In OREO, two laser pulses that represent one processing step are linked to an acoustic wave that remains inside the optical fiber much longer—because the sound velocity is 100,000x slower than the speed of light. Then, a second processing step is done via two new laser pulses and a second acoustic wave.
After the light has exited the optical fiber, both acoustic waves are still present and can interfere. “This happens for all subsequent processing steps and it means each single processing step has access to previous computational steps, which is the principle for recurrent neural networks,” Stiller explains. “Acoustics provides the recurrent functionality to the optical neural network.”
With the help of sound waves, light can be controlled with light—and it’s of interest for signal processing. “We don’t need a fabricated microstructure or transducer,” Stiller says. “The acoustic waves can be created by light waves and can, vice versa, also manipulate or better process light waves.”
A second building block is a photonic memory, which captures the important parameters of light: its phase, frequency, amplitude, pulse shape, and potentially even orbital momentum and polarization. “This information is transferred to the acoustic domain and propagates much slower, so it can act as a memory for light,” Stiller explains. “We’re now able to store a nanosecond-long pulse for about 150 ns, which is a record with acoustic waves.2 We also demonstrated short pulses down to 100 picoseconds can be stored within acoustic waves, which was predicted to be very challenging.”
And a third building block: a nonlinear activation function (currently in the preprint stage).3 “It’s an important building block because neural networks can’t be trained without nonlinearity,” Stiller points out. “Based on acoustic waves, we inserted nonlinearity into the system and experimentally showed we can create almost arbitrary nonlinear activation functions.”
Inspired by the interaction of light and traveling acoustic waves
Stiller’s work is inspired by the interaction of light and traveling acoustic waves and their features—a certain optical frequency (color of the optical wave) can only couple to a certain acoustic frequency (essentially the color of the sound wave).
Many of Stiller’s previous research works and inspiring discussions with researchers from different fields led her to try to build artificial neural networks out of optoacoustic interactions.
Perhaps not surprisingly, but interestingly, samples of optical fibers are the key ingredient in Stiller’s lab for this work. “We have a large range—from completely standard telecom fibers to toxic core fibers, microstructured fibers with tiny air channels, and soft glass fibers that have very different melting points or fiber tapers that are so thin they can’t be seen with the bare eye,” she says. “We also have glass fibers that look like fusilli pasta because they’re twisted along the propagation length.”
Pioneering a new field
To dive into a new field is a challenge of its own, Stiller acknowledges, even when it’s an extremely fascinating and motivating one. But there are fantastic upsides. “I recently had the chance to work with some very bright and interested Ph.D. and undergraduate students on this topic, so we learned a lot together and had fun,” she says. “For our optoacoustic experiments, we strive for strong interaction of optical and acoustic waves, which can be managed by different nonlinear materials, fiber structures, and also optical pulse modeling.”
Many of Stiller’s colleagues are also new to the field of neuromorphic computing and she finds it refreshing when people from different backgrounds learn together. “As far as my own work, one ‘a-ha!’ moment was that there’s so much to do in the direction of optoacoustic neural networks—the building blocks we’ve shown are just the beginning,” she says.
What’s next for Stiller’s lab? “We’ll continue to explore more complex building blocks, with a goal of building complex neural networks based on sound waves,” she says. “In the future, we envision using the same information transmission fiber for preprocessing the data as well, and ideally running a large part of the computing not within electronic motherboards but via optoacoustics within glass fibers instead.”
And she just received a European Research Council Consolidator Grant—SOUND-PC—to help her achieve this goal.
REFERENCES
- S. Becker, D. Englund, and B. Stiller, Nat. Commun., 15, 3020 (2024); https://doi.org/10.1038/s41467-024-47053-6; arXiv:2309.01543.
- A. Geilen, S. Becker, and B. Stiller, ACS Photonics (2024); https://doi.org/10.1021/acsphotonics.4c00478; arXiv:2311.06219.
- G. Slinkov, S. Becker, D. Englund, and B. Stiller, “All-optical nonlinear activation function based on stimulated Brillouin scattering,” arXiv:2401.05135 (2024).
Related Content
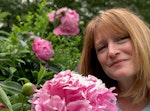
Sally Cole Johnson | Editor in Chief
Sally Cole Johnson, Laser Focus World’s editor in chief, is a science and technology journalist who specializes in physics and semiconductors. She wrote for the American Institute of Physics for more than 15 years, complexity for the Santa Fe Institute, and theoretical physics and neuroscience for the Kavli Foundation.