Novel camera calibration algorithm aims at making autonomous vehicles safer
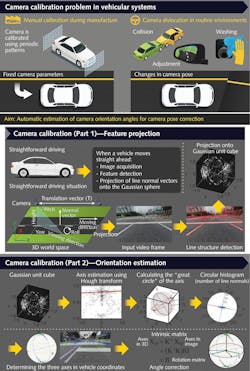
Some forms of autonomous vehicle watch the road ahead using built-in cameras. Ensuring that accurate camera orientation is maintained during driving is, therefore, in some systems key to letting these vehicles out on roads. Now, scientists from Korea have developed what they say is an accurate and efficient camera-orientation estimation method to enable such vehicles to navigate safely across distances.
Methods of estimating the orientation of cameras mounted in vehicles have been developed and advanced over the years by numerous groups of researchers. These methods have included computational approaches such as the voting algorithm, use of the Gaussian sphere, and application of deep learning and machine learning, among other techniques. However, none of these methods are fast enough to perform this estimation accurately during real time driving in real-world conditions.
To remedy the problem of estimation speed, a team of scientists from Chung-Ang University (Seoul, South Korea), led by Joonki Paik, combined some of these previously developed approaches and proposed a novel more accurate and efficient algorithm. Their method is designed for cameras with fixed focus placed at the front of the vehicle and for straightforward driving. It involves three steps. First, the image of the environment in front is captured by the camera and parallel lines on the objects in the image are mapped along the three cartesian axes. These are then projected onto what is called the Gaussian sphere, and the plane normals to these parallel lines are extracted. Second, a technique called the Hough transform, which is a feature-extraction technique, is applied to pinpoint “vanishing points” along the direction of driving. Third, using a circular histogram, the vanishing points along the two remaining perpendicular cartesian planes are identified.
Paik’s team tested this method via an experiment on roads under real driving conditions. They captured three driving environments in three videos and noted the accuracy and efficiency of the method for each. They found accurate and stable estimates in two cases. In the case of an environment captured in one of the videos, the scientists witnessed poor performance of their method because there were many trees and bushes within the camera’s range of view.
Overall, though, the method performed well under realistic driving conditions. Paik and team credit the high-speed estimation that their method can carry out to the fact that the 3D voting space is converted to a 2D plane at each step of the process.
Paik says that their method “can be immediately incorporated into automatic driver assistance systems (ADASs).” It could further be useful for a variety of alternative applications such as collision avoidance, parking assistance, and 3D map generation of the surrounding environment, thereby preventing accidents and promoting safer driving environments. “We are planning to extend this to smartphone applications like augmented reality and 3D reconstruction,” he adds.
Source: Chung-Ang University
REFERENCE:
1. Youngran Jo et al., Optics Express (2019); https://doi.org/10.1364/OE.27.026600.
Got optics- and photonics-related news to share with us? Contact John Wallace, Senior Editor, Laser Focus World
Get more like this delivered right to your inbox
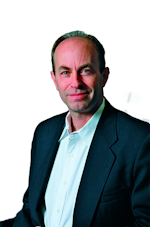
John Wallace | Senior Technical Editor (1998-2022)
John Wallace was with Laser Focus World for nearly 25 years, retiring in late June 2022. He obtained a bachelor's degree in mechanical engineering and physics at Rutgers University and a master's in optical engineering at the University of Rochester. Before becoming an editor, John worked as an engineer at RCA, Exxon, Eastman Kodak, and GCA Corporation.