Shining expertly timed lasers at an approaching autonomous vehicle’s LiDAR system can create a large blindspot in front of it—hiding moving pedestrians and other obstacles.
This security vulnerability, which tricks LiDAR sensors into deleting data about pedestrians and obstacles, was discovered by a group of researchers from the University of Florida, University of Michigan, and University of Electro-Communications in Japan (see Fig. 1 and video). Thankfully, they also provide upgrades/fixes to protect LiDAR sensors from malicious attacks.
“In my cyber-physical security system lab, we investigate the trustworthiness and security of sensors when used for executing automatic critical operations,” says Sara Rampazzi, a professor in the Department of Computer and Information Science and Engineering at the University of Florida. “Autonomous driving technology’s evolving at a rapid pace, but we observed from the user’s experience how the vehicle perception, namely what the car ‘sees’ with its sensors—cameras and LiDARs—can be misinterpreted due to natural changes within the environment.”
As a not-too-long-ago example, Rampazzi points to Tesla’s full self-driving feature mistaking the moon for a yellow traffic light. Exploring more sinister situations where malicious attackers can actively manipulate what the car sees to intentionally induce these misinterpretations and how they can help solve these problems is the inspiration behind her group’s work.
“There’s a fundamental knowledge gap between what a system can perceive from the surroundings and what humans ‘expect’ the system to perceive,” says Rampazzi. “Blindly relying on data coming from sensors to perform critical decisions humans make about steering or braking to avoid a collision can have unexpected consequences. For this reason, it’s paramount to study the underlying technology and potential vulnerabilities to build secure and safe technology we can trust.”
Exploiting LiDAR sensors
LiDAR sensors work by “firing laser pulses and calculating the distance from the sensor and potential obstacles in the car trajectory, based on the timing of returned signals,” says Yulong Cao, a Ph.D. student at the University of Michigan. “Due to the noisy signals returned in the real world, LiDAR sensors used in autonomous vehicles usually prioritize the first/strongest signals received, and filter out signals returned too soon, a.k.a. close reflections.”
Attackers can “exploit this designed behavior to induce the automatic discard of the returned signals coming from real pedestrians or cars in the road far away, by generating laser pulses to mimic fake returned signals closer to the LiDAR sensor,” says Rampazzi. “Since these fake signals are then automatically filtered out by the sensor as well, the attack remains completely unnoticed and real obstacles aren’t perceived anymore.”
The LiDAR still receives genuine data from the obstacle, but the data gets automatically discarded because the group’s fake reflections are the only ones the sensor perceives.
Putting it to the test
To demonstrate the attack, the group used moving vehicles and robots with the attacker device located on the side of the road about 15 feet away. But, in theory, this can be accomplished from farther away with fancier equipment.
The tech involving in pulling this off is pretty basic, but the laser must be perfectly timed to the LiDAR sensor and moving vehicles must be carefully tracked to keep the laser pointing in the right direction. All the information you need is “usually publicly available from the manufacturer,” says S. Hrushikesh Bhupathiraj, a Ph.D. student at the University of Florida.
Thanks to this technique, the group managed to delete data for static obstacles and moving pedestrians (see Fig. 2). They also demonstrated with real-world experiments how attackers could follow a slow-moving vehicle using basic camera tracking equipment.In their simulations of autonomous vehicle decision-making, the deletion of data caused a car to continue accelerating toward a pedestrian it could no longer see—instead of stopping.
“The most exciting aspect of our work is we discovered an attacker can selectively remove an entire area of the LiDAR field-of-view, while the spoofed signals go fully unnoticed by the system,” says Bhupathiraj.
And the most challenging part was “identifying the cause of the LiDAR behavior, especially because we analyze the sensors as a black box for proprietary reasons,” Bhupathiraj adds. “Raw LiDAR data goes through multiple layers of processing, and the filtering can take place at any of the layers. Another challenge was realizing the attack aiming the laser on a moving vehicle while being able to stably spoof the fake reflections to the LiDAR.”
Fixes for LiDAR manufacturers
Updates to the LiDAR sensors or software that interpret the raw data should fix this security vulnerability, and the researchers recommend manufacturers start teaching software to look for the telltale signatures of spoofed reflections added by a laser attack.
“We hope our discovery and our proposed strategies to overcome this vulnerability will help LiDAR manufacturers and autonomous driving frameworks designers build a new generation of self-driving technology, more resilient to these attacks that remain mostly overlooked,” Rampazzi says.
The group is continuing to explore sensor technologies to understand how attackers can take advantage of these systems to undermine the security and safety of autonomous vehicles. “Our goal is to provide solutions to these problems and help advance autonomous driving technology,” says Rampazzi.
FURTHER READING
See www.usenix.org/system/files/sec23summer_349-cao-prepub.pdf.
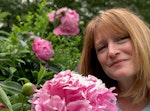
Sally Cole Johnson | Editor in Chief
Sally Cole Johnson, Laser Focus World’s editor in chief, is a science and technology journalist who specializes in physics and semiconductors. She wrote for the American Institute of Physics for more than 15 years, complexity for the Santa Fe Institute, and theoretical physics and neuroscience for the Kavli Foundation.